The Ultimate Guide to Semantic Segmentation Video
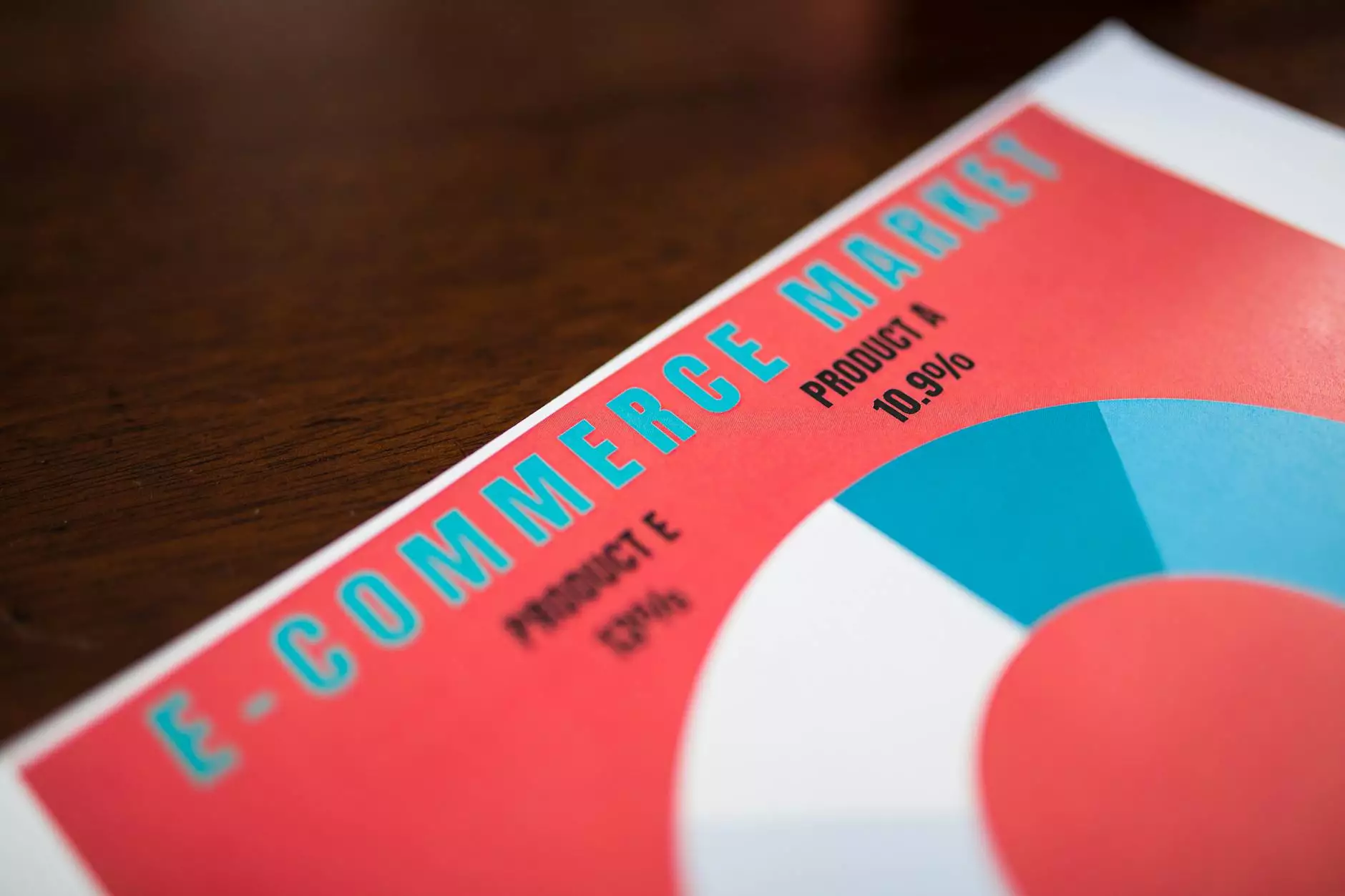
Semantic segmentation video plays a crucial role in the rapidly evolving field of artificial intelligence and computer vision. By segmenting each pixel of a video into distinct classes, systems can perceive and interpret scenes much like a human does. At KeyLabs.ai, we harness the power of advanced data annotation tools and platforms to revolutionize how businesses leverage video data. In this detailed guide, we will explore the various aspects of semantic segmentation in video, how it is applied across industries, and how to utilize data annotation tools effectively.
Understanding Semantic Segmentation
Semantic segmentation is a form of image analysis where each pixel in an image (or video frame) is classified into meaningful categories. Unlike traditional segmentation methods, which might only create bounding boxes around objects, semantic segmentation provides a more granular understanding of the environment.
The Importance of Semantic Segmentation in Video
With the explosion of video content across platforms, semantic segmentation allows machines to comprehend scenes in a way that enables various applications:
- Autonomous Vehicles: Semantic segmentation helps in identifying lanes, pedestrians, vehicles, and traffic signs, enhancing navigation systems.
- Healthcare: In the medical field, it can assist in identifying tumors and other significant structures in 3D medical imaging.
- Smart City Management: Analyzing urban dynamics can improve traffic flow and public safety measures.
- Retail Analytics: Understanding customer behavior through in-store video analysis helps tailor marketing strategies effectively.
How Semantic Segmentation Works
The process of semantic segmentation involves several key steps:
1. Data Collection
The first step is to collect a diverse dataset of video footage. This data should encompass a variety of environments, lighting conditions, and scenarios to ensure the model's robustness.
2. Data Annotation
Data annotation is crucial for training convolutional neural networks (CNNs). Each frame in the video must be meticulously annotated to label every pixel accurately. This is where high-quality data annotation tools come into play. At KeyLabs.ai, our data annotation platform provides state-of-the-art tools that allow for precise and efficient annotation.
3. Training the Model
Once annotated, the dataset is used to train models like U-Net, Fully Convolutional Networks (FCNs), or DeepLab. These models learn to recognize patterns and features within the labeled data to produce accurate segmentation maps for new, unseen video content.
4. Validation and Testing
The trained model goes through validation and testing phases to evaluate its accuracy and performance. Metrics such as Intersection over Union (IoU) are commonly used to assess how well the model segments objects in video.
Best Practices in Semantic Segmentation Video Projects
To achieve success in projects involving semantic segmentation of videos, consider the following best practices:
1. Utilize High-Quality Annotation Tools
Choosing a reliable data annotation tool is pivotal. KeyLabs.ai offers intuitive interfaces and features that enhance productivity while ensuring accuracy.
2. Ensure Diverse Dataset
A diverse dataset helps the model generalize better. Include videos from different domains, settings, and conditions.
3. Implement Regular Updates
As new data becomes available, regularly update your model with fresh annotations to maintain accuracy over time.
4. Leverage Data Augmentation
Augmenting your dataset with transformations like rotation, scaling, and flipping can improve model robustness by simulating various real-world conditions.
Applications of Semantic Segmentation in Industry
Semantic segmentation in video has transformative applications across several industries:
1. Automotive Industry
In autonomous driving, real-time semantic segmentation analyzes roads, vehicles, and pedestrians. It helps cars make informed decisions while navigating complex environments.
2. Surveillance and Security
Semantic segmentation can enhance surveillance systems by identifying suspicious behavior or recognizing individuals in various environments, contributing to improved safety measures.
3. Augmented Reality (AR) and Virtual Reality (VR)
In AR and VR, semantic segmentation helps create immersive environments where virtual elements can seamlessly interact with the real world.
4. Environmental Monitoring
In ecology, it enables tracking wildlife and vegetation health by analyzing aerial or ground videos, aiding conservation efforts and environmental studies.
The Future of Semantic Segmentation in Video
As technology progresses, the capabilities of semantic segmentation continue to evolve. Future developments may include:
- Real-time processing: Enhanced algorithms and hardware improvements may allow for faster, real-time segmentation in high-definition video streams.
- Integration with AI: Combining semantic segmentation with AI decision-making systems could lead to more autonomous solutions across industries.
- Increased accuracy: Research in deep learning is ongoing, promising even higher segmentation accuracy and efficiency.
- Broader applications: Expanding use cases in sectors like entertainment, sports analysis, and more personalized user experiences.
Conclusion
In conclusion, the integration of semantic segmentation video in artificial intelligence frameworks marks a significant advancement in how machines interpret and interact with the world. As illustrated throughout this guide, utilizing comprehensive data annotation tools like those offered by KeyLabs.ai is essential for organizations looking to harness the full potential of their video data.
By adopting best practices and understanding the intricate processes involved in semantic segmentation, businesses can leverage this technology for innovative applications that improve operational efficiencies and enhance the user experience. The future of semantic segmentation holds immense promise, paving the way for smarter, more responsive systems across various industries.